گزارشی از چالش استدلال و انتزاع ـ سایت کَگل
Abstraction and Reasoning Challenge - This competition was hosted by François Chollet
- این گزارش توسط سمیه غلامی و مهران کاظمی نیا تهیه شده است.
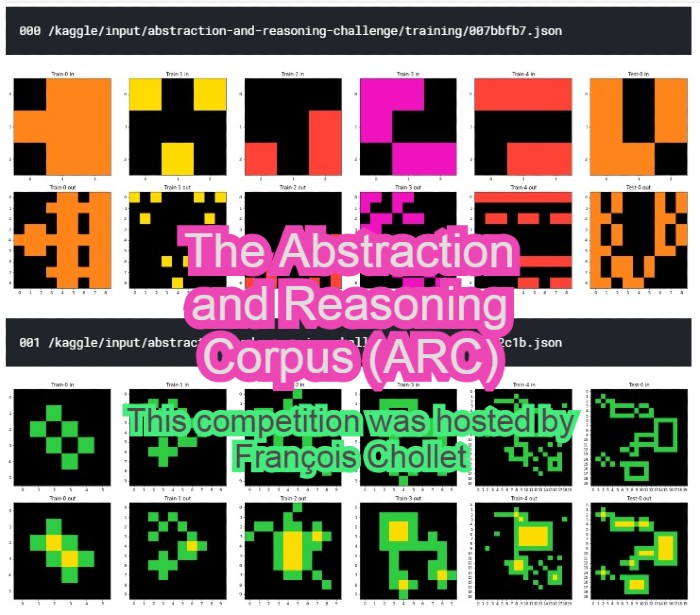
درحال حاضر، تکنیک های یادگیری ماشین فقط می توانند از الگوهای که قبلا دیده اند، استفاده کنند. یعنی از ابتدا برای ماشین ها، الگوهای مشخصی تعیین می شود و سپس درمعرض داده های مرتبط قرار می گیرند، تا بتوانند مهارت های جدیدی بیاموزند. ولی آیا درآینده ، ماشین ها می توانند مانند انسان ها، به سوالاتِ استدلالی که تا به حال ندیده اند، پاسخ صحیح بدهند؟ آیا ماشین ها می توانند کارهای پیچیده و انتزاعی را فقط از چند نمونه بیاموزند؟ این دقیقا موضوع مسابقه استدلال و انتزاع بود که اخیرا به پایان رسید و یکی از بحث بر انگیزترین چالش های کَگل نیز محسوب می شود. در این چالش از شرکت کنندگان خواسته شد تا یک هوش مصنوعی را در مدت سه ماه ایجاد کنند، تا بتواند سوالاتِ استدلالی را که قبلاً ندیده است، حل کند. کگل در معرفی این مسابقه نوشته است:
"It provides a glimpse of a future where AI could quickly learn to solve new problems on its own. The Kaggle Abstraction and Reasoning Challenge invites you to try your hand at bringing this future into the present!"
سوالات استدلالی این چالش، مانند آزمون های هوش برای انسان ها بود و سوالات ساده، متوسط و گاهی نسبتا سخت را شامل می شد. البته یک انسان معمولی قادر بود، همه سوالات را در یک زمان معقول، پاسخ دهد و هیچکدام از سوالات، پیچیدگی خارق العاده ای نداشت. ولی چالش این بود که همه مفاهیم استدلالی مثل؛ تغییر رنگ، تغییر سایز، تغییر در ترتیب قرار گیری و … را چگونه می توان به ماشین آموزش داد تا ماشین هم بتواند در یک آزمون هوش انسانی، که تا به حال ندیده است، موفقیت کسب کند.
جایزه این مسابقه مجموعا مبلغ بیست هزار دلار بود که بین سه نفر اول (سه تیم اول) تقسیم شد. اما همانطورکه حدس زده می شد؛ حتی نتایج نفرات اول نیز، امیدوارکننده نبود. این چالش تقریبا هزار شرکت کننده داشت که الگوریتم های نیمی از آنها به هیچ کدام از سوالات پاسخ صحیح ندادند. اگر الگوریتم یک تیم اصلا کار نمی کرد، امتیاز یک می گرفت و اگر می توانست به تعداد کمی از سوالات، پاسخ صحیح بدهد، مثلا امتیاز نود و هشت صدم یا … دریافت می کرد. به هرحال فقط دوازده تیم توانستند کمتر از نود صدم امتیاز بگیرند. در ادامه جدول نهایی امتیازات مسابقه برای سی نفر اول، آورده شده است.
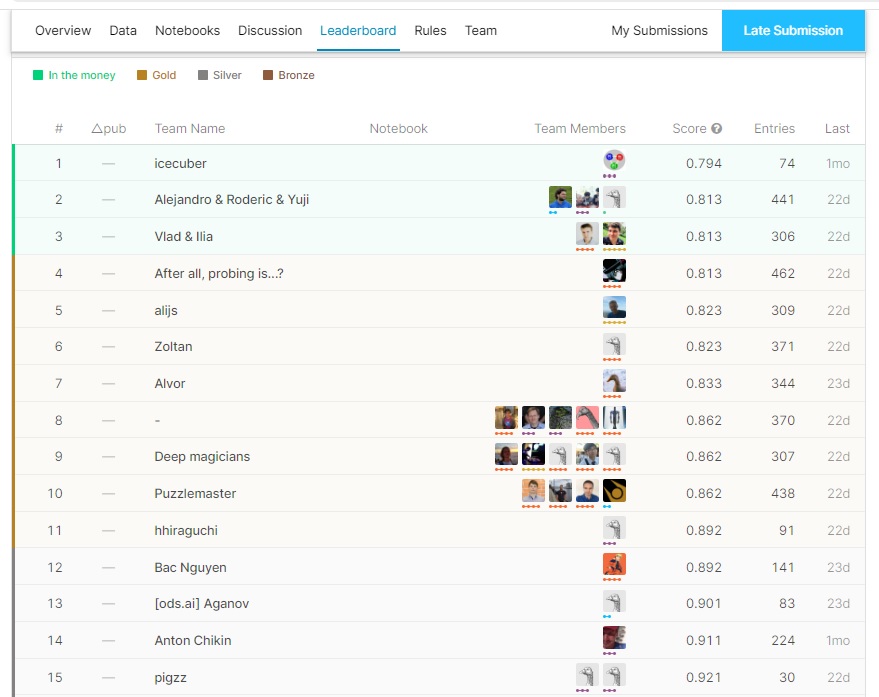
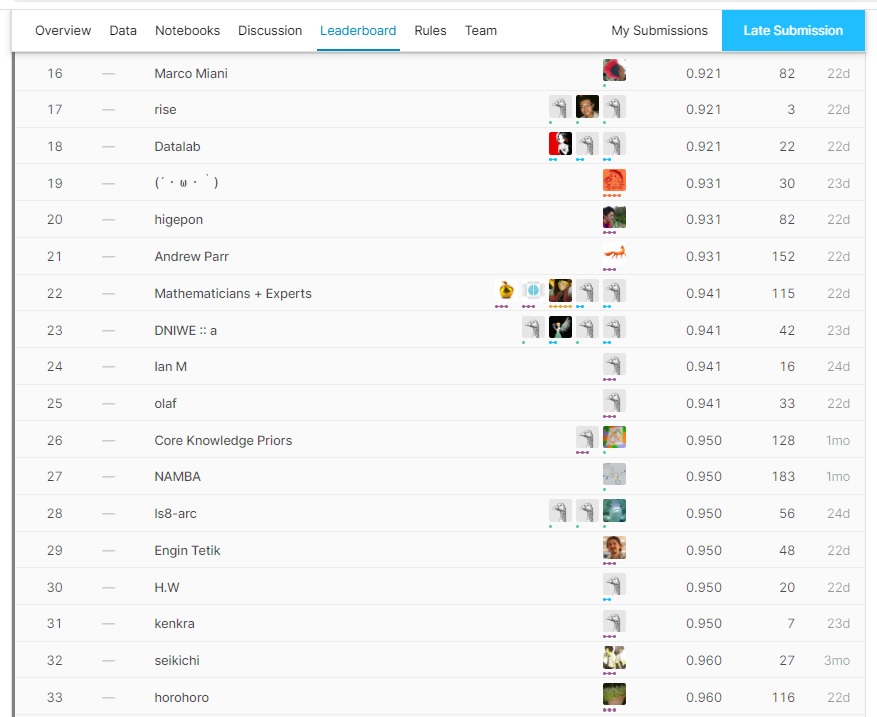
این مسابقه یک چالش کلاس بندی نبود، یعنی جواب ها از بین چند گزینه تصویری انتخاب نمی شدند. بلکه همه جواب ها می بایست بصورت تصویر(ماتریس) ساخته می شدند. این موضوع ، مسابقه را پیچیده تر می کرد. شاید به همین دلیل، کسانی که فکر می کردند می توانند صرفا با روش های مرسوم و کلاسیک، ماشین را آموزش دهند و یا با حدس و گمان، کار را جلو ببرند، کاملا مایوس شدند. البته بعضی از شرکت کنندگان به حل نمونه هایی پرداختند که راه حل های ساده تری داشتند و به نوعی استثناء محسوب می شدند. طبیعی است که این افراد در بهترین حالت فقط تعداد کمی از نمونه ها را حل نمودند و موفقیت زیادی کسب نکردند.
با این که حقیقتا نبوغ و تلاش برندگان و شرکت کنندگان این مسابقه قابل تحسین است، ولی با یک نگاه به جدول امتیازات، به نظر می رسد که هنوز با جواب نهایی فاصله داریم و تضمینی نیست که بهترین روش ها، توسط شرکت کنندگان انتخاب شده باشد. به هرحال برندگان این مسابقه با سخاوتمندی، روش خلاقانه خود را در لینک های زیر شرح داده اند و برخی از آنها کدهای کامل خود را نیز ارائه کرده اند.
List of gold medal solutions shared:
1st place solution by icecuber
2nd place solution by Alejandro de Miquel
3rd place solution by Vlad Golubev
3rd place solution by Ilia
5th place solution by alijs
6th place solution by Zoltan
8th place solution by Andy Penrose
8th place solution by Maciej Sypetkowski
8th place solution by Jan Bre
9th place solution by Hieu Phung
10th place solution by Alexander Fritzler
اگر شما به این موضوع علاقه مند شدید، می توانید روی سایت کَگل و همچنین گیت هاب فرانسوا شله ، اطلاعات بسیار زیادی را از این چالش کسب کنید. البته اگر بخواهید ابتکار خود را داشته باشید و راه حل های خود را امتحان کنید ، چند توصیه برای شما داریم ؛ برای شروع کار ابتدا مقاله ۶۴ صفحه ای آقای فرانسوا شله در ارتباط با اندازه گیری هوش را بخوانید:
On the Measure of Intelligence | François Chollet
ضمنا در سایت کگل می توانید به بخش Discussion و Notebooks این چالش رجوع کنید و مستقیما توصیه های میزبان، برندگان و همه شرکت کنندگان را مطالعه کنید. در انتها، توجه شما را به چند توصیه کلیدی از فرانسوا شله جلب می کنیم:
How to get started?
fchollet - Competition Host:
If you don't know how to get started, I would suggest the following template:
Take a bunch of tasks from the training or evaluation set -- around 10.
For each task, write by hand a simple program that solves it. It doesn't matter what programming language you use -- pick what you're comfortable with.
Now, look at your programs, and ponder the following:
1) Could they be expressed more naturally in a different medium (what we call a DSL, a domain-specific language)?
2) What would a search process that outputs such programs look like (regardless of conditioning the search on the task data)?
3) How could you simplify this search by conditioning it on the task data?
4) Once you have a set of generated candidates for a solution program, how do you pick the one most likely to generalize?
You will not find tutorials online on how to do any of this. The best you can do is read past literature on program synthesis, which will help with step 3). But even that may not be that useful :)
This challenge is something new. You are expected to think on your own and come up with novel, creative ideas. It's what's fun about it!
Does hard-coding rules disqualify?
fchollet - Competition Host:
You can hard-code rules & knowledge, and you can use external data
Can we "probe" the leaderboard to get information about the test set?
fchollet - Competition Host:
Using your LB score as feedback to guess the exact contents of the test set is against the spirit of the competition. In fact, it is against the spirit of every Kaggle competition. The goal of the competition is to create an algo that will turn the demonstration pairs of a task into a program that solves the task -- not to reverse-engineer the private test set.
Further, this is a waste of your time. It is extremely unlikely that you would be able to guess an exact output or an exact task. This is why we decided not to have a separate public and private leaderboard: probing is simply not going to work.
That is because:
1) test tasks have no exact overlap with training and eval tasks (although they look "similar" in the sense that they're the same kind of puzzle, built on top of Core Knowledge systems)
2) the space of all possible ARC tasks is very large, and very diverse.
So you're not going to get a hit by either trying everything found in the train and eval set, or by just randomly guessing new tasks. You would have better luck trying to guess the exact melodies of the top 100 pop songs of 2021.
Is the level of difficulty similar in evaluation set and test set?
fchollet - Competition Host:
The difficulty level of the evaluation set and test set are about the same. Both are more difficult than the training set. That is because the training set deliberately contains elementary tasks meant to serve as Core Knowledge concept demonstration.
Can we use data from both the training and evaluation sets in our solutions?
fchollet - Competition Host:
I would recommend only using data from the training set to develop your algorithm. Using data from both the training set and evaluation set isn't at all against the rules, so could you do it, but it would be bad practice, since it would prevent you from accurately evaluating your algorithms.
The goal of this competition is to develop an algorithm that can make sense of tasks it has never seen before. You'll want to be able to check how well your algorithm perform before submitting it. For this purpose, you need a set of tasks that your algorithm has never seen, and further, that you have never seen. That's the evaluation set. So don't leak too much into information from the evaluation set into your algorithm, or you won't be able to evaluate it.
Note that the "test" set is a placeholder (copied from the evaluation set) for you to check that your submission is working as intended. The real test set used for the leaderboard is fully private.
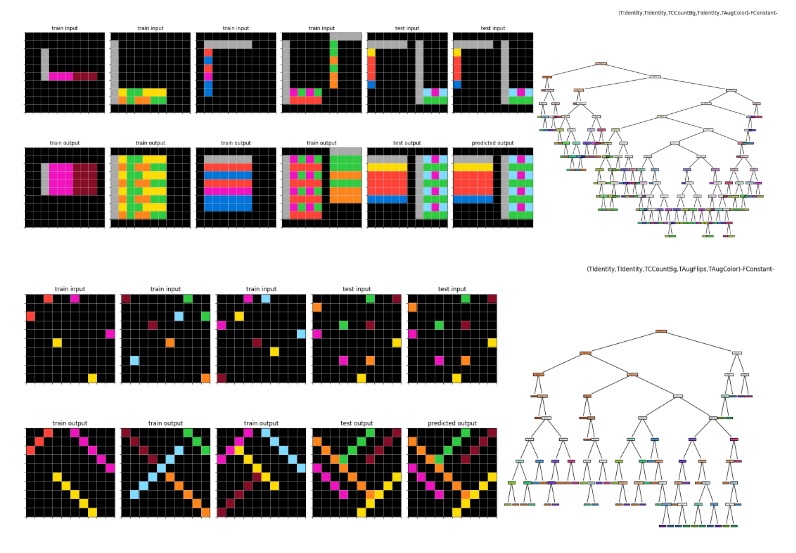
پس همه چیز آماده است.
یک قهوه بنوشید و کار خود را شروع کنید.
موفق باشید.
سمیه غلامی و مهران کاظمی نیا
مطلبی دیگر از این انتشارات
Cognitive Computing - محاسبات شناختی
مطلبی دیگر از این انتشارات
اینستاگرام و نگاه ِ داییجانناپلئونی به آن
مطلبی دیگر از این انتشارات
یادگیری ماشین (بخش اول) - معرفی